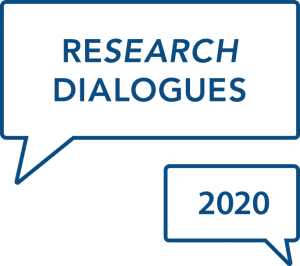
Day 2, April 15 - Posters
Start Date
15-4-2020 1:00 PM
End Date
15-4-2020 3:00 PM
Publisher
University of Tennessee at Chattanooga
Place of Publication
Chattanooga (Tenn.)
Abstract
SETs are analyzed and characterized through ionizing radiation effects spectroscopy (IRES) and machine learning. Potentially catastrophic radiation-induced errors can be exposed with IRES as it simplifies the identification of transients through statistical analysis of waveform behavior, allowing for the capture of subtle changes in circuit dynamics. Leveraging a k-Nearest Neighbors (KNN) machine learning algorithm with IRES data, the identification of transients is facilitated and makes an on-chip implementation feasible.
Date
4-15-2020
Document Type
posters
Language
English
Rights
http://rightsstatements.org/vocab/InC/1.0/
License
http://creativecommons.org/licenses/by/4.0/
Recommended Citation
Cancelleri, Joseph, "Detecting and Identifying Single Event Transients using IRES and Machine Learning". ReSEARCH Dialogues Conference proceedings. https://scholar.utc.edu/research-dialogues/2020/day2_posters/110.
Detecting and Identifying Single Event Transients using IRES and Machine Learning
SETs are analyzed and characterized through ionizing radiation effects spectroscopy (IRES) and machine learning. Potentially catastrophic radiation-induced errors can be exposed with IRES as it simplifies the identification of transients through statistical analysis of waveform behavior, allowing for the capture of subtle changes in circuit dynamics. Leveraging a k-Nearest Neighbors (KNN) machine learning algorithm with IRES data, the identification of transients is facilitated and makes an on-chip implementation feasible.